In the ever-evolving field of medicine, the integration of digital health technology is paving the way for a new era of personalized medicine. With the ability to collect vast amounts of individualized health data, analyze it in real-time, and deliver tailored treatment plans, digital health is revolutionizing patient care. This article explores the profound impact that digital technology is having on the future of personalized medicine, providing insight into the potential benefits, challenges, and ethical considerations that arise from this exciting advancement.
Table of Contents
Current Landscape of Personalized Medicine
Advancements in Genomics
Advancements in genomics have revolutionized the field of personalized medicine. The ability to sequence an individual’s genome has provided valuable insights into their genetic makeup and susceptibility to various diseases. Genomic sequencing techniques, such as next-generation sequencing, have become more affordable and offer reduced turnaround times, making it easier to incorporate genomics into clinical practice. This has paved the way for targeted therapies and personalized treatment plans tailored to an individual’s unique genetic profile.
Integration of Machine Learning
The integration of machine learning in personalized medicine has further accelerated the progress in this field. Machine learning algorithms can analyze vast amounts of genomic and patient data, uncover patterns, and make predictions. This enables healthcare professionals to identify genetic markers associated with specific diseases, predict disease outcomes, and develop personalized treatment plans. Machine learning has the potential to greatly enhance diagnostic accuracy and treatment effectiveness, improving patient outcomes in the process.
Emerging Technologies in Digital Health
Digital health technologies are playing a crucial role in moving personalized medicine forward. Emerging technologies, such as wearable devices and remote monitoring, allow for continuous data collection and monitoring of patients’ health parameters. Real-time health monitoring and the use of digital biomarkers enable early detection of changes in physiological and behavioral patterns, allowing for timely intervention and disease management. These technologies empower patients to actively participate in their healthcare journey and make informed decisions regarding their treatment options.
Role of Digital Health in Personalized Medicine
Data Collection and Analysis
Digital health enables the comprehensive collection and analysis of patient data, including genomic information, medical records, lifestyle factors, and environmental data. This holistic approach to data collection allows healthcare professionals to gain a deeper understanding of individual patients and develop personalized treatment strategies. By leveraging digital health technologies, healthcare providers can identify patterns, predict disease progression, and assess treatment response more accurately.
Improved Diagnosis and Treatment
The integration of digital health in personalized medicine has revolutionized the diagnostic process. By analyzing patient data using machine learning algorithms, healthcare professionals can generate accurate and timely diagnoses. This leads to more precise treatment plans tailored to the individual patient, resulting in improved outcomes. Digital health tools also enable the monitoring of treatment effectiveness, allowing for adjustments and refinements over time, ultimately leading to better patient care.
Patient Empowerment and Engagement
Digital health technologies have created opportunities for patients to actively engage in their own healthcare. Through access to personal health data, individuals can monitor their health status, track progress, and gain insights into their own well-being. This empowerment promotes a sense of ownership and responsibility for one’s health, leading to improved health outcomes. Additionally, digital health facilitates shared decision-making between healthcare providers and patients, ensuring that treatment plans align with the patient’s values and preferences.
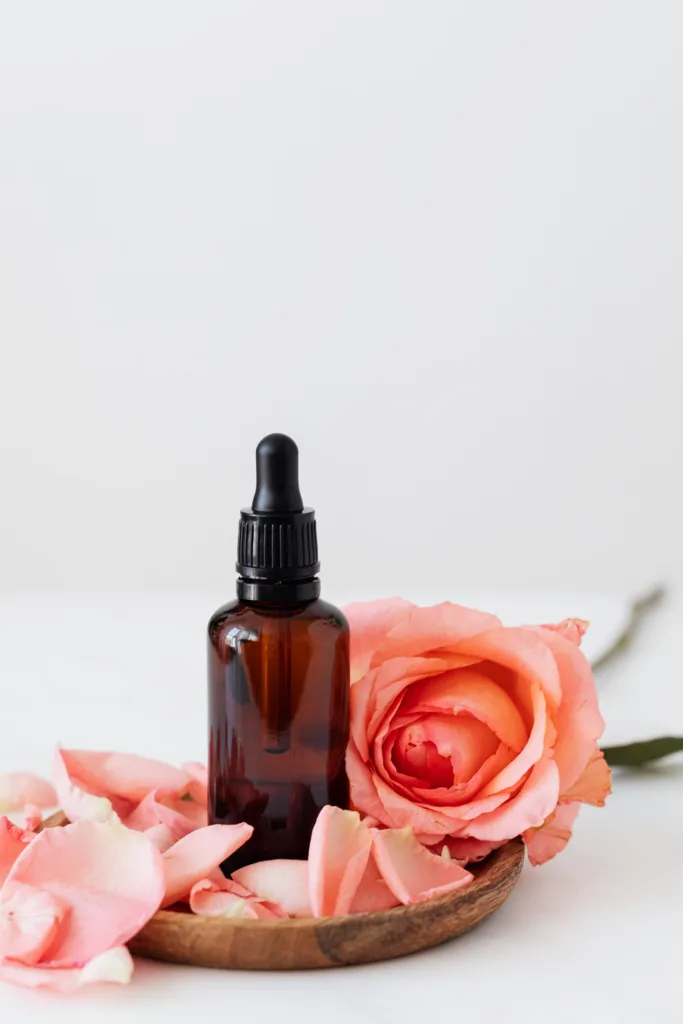
Challenges in Implementing Personalized Medicine through Digital Health
Privacy and Security Concerns
As the use of digital health technologies expands, privacy and security concerns become more prominent. The extensive collection, storage, and analysis of patient data raise ethical and legal considerations. Safeguarding patient privacy and ensuring the secure transmission of sensitive information are crucial to building trust in personalized medicine. Regulatory frameworks must be in place to protect patient data and ensure compliance with privacy regulations.
Regulatory Hurdles
Regulatory challenges pose significant barriers to the implementation of personalized medicine through digital health. The landscape of healthcare regulations and policies varies across different jurisdictions, making it difficult to establish standardized protocols for data sharing and analysis. Streamlining regulatory processes and harmonizing standards can facilitate the effective integration of digital health solutions into clinical practice, fostering the advancement of personalized medicine.
Interoperability and Standardization
Interoperability and standardization are key challenges in implementing personalized medicine through digital health. Healthcare systems often utilize different electronic medical record (EMR) systems that may not communicate seamlessly with each other. This lack of interoperability inhibits the efficient exchange of patient data, hindering comprehensive analysis and personalized treatment planning. Standardization efforts are required to overcome these obstacles and create a cohesive digital infrastructure that supports personalized medicine.
Advancements in Genomic Sequencing
Next-Generation Sequencing Techniques
Advancements in genomic sequencing techniques have drastically transformed personalized medicine. Next-generation sequencing (NGS) allows for the rapid, accurate, and cost-effective analysis of a patient’s entire genome or specific genetic regions. NGS techniques have overcome the limitations of traditional sequencing methods, enabling widespread genomic profiling for personalized medicine practices. The ability to analyze a large number of genes simultaneously has greatly enhanced our understanding of genetic variations and their association with diseases.
Reduced Cost and Turnaround Time
The cost of genomic sequencing has significantly declined over the years, making it more accessible to healthcare providers and patients. The reduced cost has democratized personalized medicine, allowing more individuals to benefit from genomic analysis and targeted therapies. Additionally, advancements in genomic sequencing techniques have led to decreased turnaround times, enabling healthcare professionals to make timely and informed decisions regarding patient care. The affordability and efficiency of genomic sequencing are crucial factors in driving the adoption of personalized medicine.

Integration of Artificial Intelligence in Personalized Medicine
Machine Learning Algorithms for Data Analysis
Artificial intelligence (AI), specifically machine learning, has revolutionized data analysis in personalized medicine. Machine learning algorithms can sift through vast amounts of patient data, including genomic information, medical records, and other relevant datasets, to uncover patterns and associations. This analysis can identify genetic markers, predict disease outcomes, and recommend personalized treatment strategies. Machine learning algorithms continually learn and improve from new data, ensuring that personalized medicine practices keep pace with the evolving field of healthcare.
AI-driven Diagnosis and Treatment
AI-powered systems have the potential to enhance diagnostic accuracy and treatment outcomes in personalized medicine. By comparing patient data to vast databases of medical knowledge, AI algorithms can provide accurate and timely diagnoses. AI-driven systems can assist healthcare professionals in identifying optimal treatment strategies and predicting treatment responses based on the patient’s unique characteristics. This integration of AI in personalized medicine holds great promise for improving patient outcomes and transforming healthcare delivery.
Wearable Devices and Remote Monitoring
Continuous Data Collection and Monitoring
Wearable devices, such as fitness trackers and smartwatches, enable the continuous collection of various health parameters, including heart rate, sleep patterns, physical activity levels, and more. This continuous data collection provides healthcare professionals with a comprehensive picture of the patient’s overall health and well-being. The ability to monitor these parameters in real-time allows for early detection of deviations from the norm, alerting healthcare providers to potential health concerns and enabling timely intervention.
Real-time Health Monitoring
Real-time health monitoring through wearable devices allows for proactive healthcare management. By tracking physiological and behavioral parameters, such as blood pressure, glucose levels, and daily activity, healthcare professionals can detect early signs of health issues and make informed decisions regarding treatment plans. Real-time health monitoring also promotes patient engagement, as individuals can actively participate in their health management by setting goals, tracking progress, and making lifestyle modifications based on the insights provided by wearable devices.
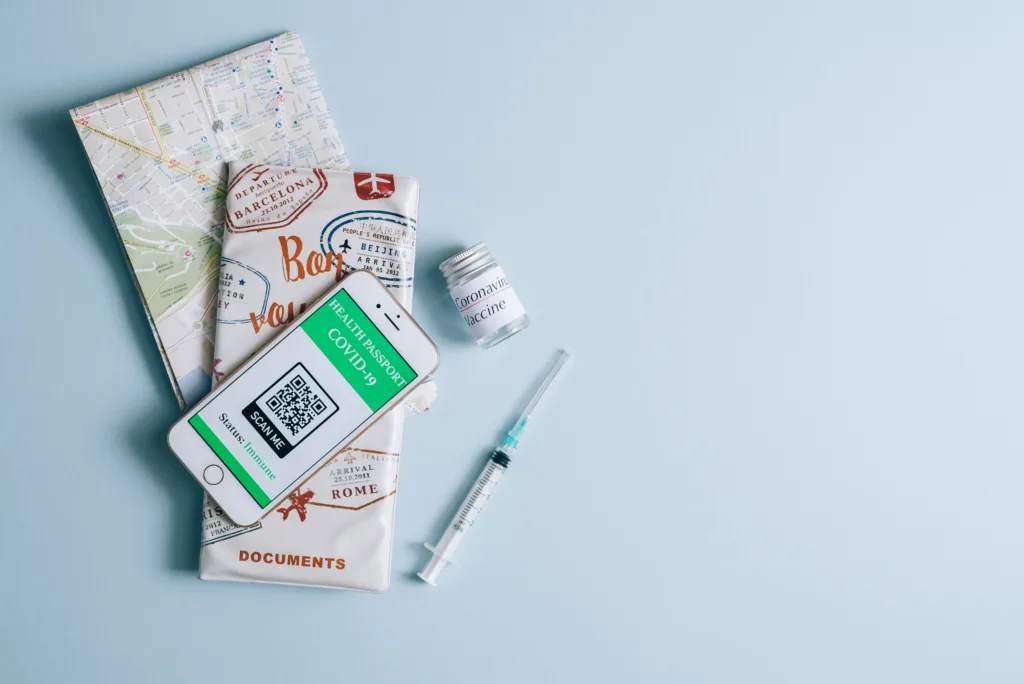
Digital Biomarkers for Disease Monitoring
Tracking Physiological and Behavioral Parameters
Digital biomarkers offer a vast array of opportunities for disease monitoring and management. These biomarkers capture various physiological and behavioral parameters, such as heart rate variability, sleep quality, medication adherence, and emotional well-being. By continuously tracking these parameters through digital health technologies, healthcare professionals can identify patterns of disease progression or treatment response. Digital biomarkers provide valuable insights into an individual’s health status and can facilitate personalized treatment adjustments.
Predictive Analysis for Disease Progression
Through the analysis of digital biomarkers, predictive models can be created to forecast disease progression and identify potential complications. Machine learning algorithms can analyze large datasets from multiple patients to identify patterns and trends indicative of disease outcomes. Predictive analysis can help healthcare professionals assess the risk of disease progression and develop personalized treatment strategies to mitigate these risks. The integration of digital biomarkers and predictive analysis holds great potential for optimizing disease management and improving patient outcomes.
Enhanced Diagnosis and Treatment through Digital Health
Improved Disease Risk Assessment
Digital health technologies enable healthcare professionals to obtain a more comprehensive understanding of an individual’s disease risk. By combining genomic data, medical history, lifestyle factors, and environmental exposures, healthcare providers can accurately assess an individual’s likelihood of developing certain diseases. This knowledge allows for targeted interventions, including preventive measures and early screening, to reduce disease burden and improve outcomes. Improved disease risk assessment through digital health empowers individuals to take proactive steps towards maintaining their health.
Precision Medicine Approaches
Digital health tools support the implementation of precision medicine approaches in personalized medicine. Precision medicine aims to deliver the right treatment to the right patient at the right time, considering their individual genetic profile, environmental influences, and lifestyle factors. By integrating digital health technologies, healthcare professionals can identify the most effective treatment options for the individual patient, reducing the risk of adverse reactions and improving treatment outcomes. Precision medicine approaches hold great promise for tackling complex diseases and tailoring treatment plans based on individual needs.

Patient Empowerment and Engagement
Access to Personal Health Data
Digital health tools provide patients with access to their personal health data, empowering them to take an active role in managing their health. By accessing their medical records, test results, and treatment plans, patients can gain a greater understanding of their health status and make informed decisions about their care. This transparency fosters engagement and collaboration between patients and healthcare providers, ultimately leading to improved health outcomes and patient satisfaction.
Shared Decision-Making
Digital health promotes shared decision-making between healthcare providers and patients. Through digital platforms and mobile applications, patients can actively participate in their treatment decisions and be involved in setting goals and evaluating treatment options. Shared decision-making takes into account the patient’s preferences, values, and personal circumstances, ensuring that treatment plans align with their individual needs. This collaborative approach enhances patient satisfaction, improves treatment adherence, and leads to better overall health outcomes.
Interoperability and Standardization Challenges
Data Exchange among Healthcare Systems
Interoperability challenges hinder the efficient exchange of patient data among different healthcare systems. Lack of standardized formats and systems makes it difficult to seamlessly share and integrate patient information. This poses a significant barrier to personalized medicine, as comprehensive analysis and treatment planning rely on access to a patient’s complete medical history and relevant data. Establishing standardized protocols for data exchange and interoperability is crucial for harnessing the full potential of digital health in personalized medicine.
Integration of Digital Health Solutions
The integration of digital health solutions into existing healthcare systems is often fragmented and challenging. Healthcare systems use different electronic medical record (EMR) systems that may not communicate seamlessly with each other. This lack of integration inhibits the efficient sharing and analysis of patient data, hindering personalized treatment planning. Standardization efforts are necessary to overcome these interoperability challenges and create a unified digital infrastructure that supports the implementation of personalized medicine through digital health.
In conclusion, personalized medicine has made significant strides with the integration of digital health technologies. Advancements in genomics, the integration of machine learning, and the emergence of wearable devices and remote monitoring have transformed the landscape of personalized medicine. These technologies enable comprehensive data collection, analysis, and personalized treatment planning. However, challenges such as privacy concerns, regulatory hurdles, and interoperability issues must be addressed to fully harness the potential of personalized medicine through digital health. With continued advancements and collaboration, the future of personalized medicine holds great promise in improving patient outcomes and revolutionizing healthcare delivery.
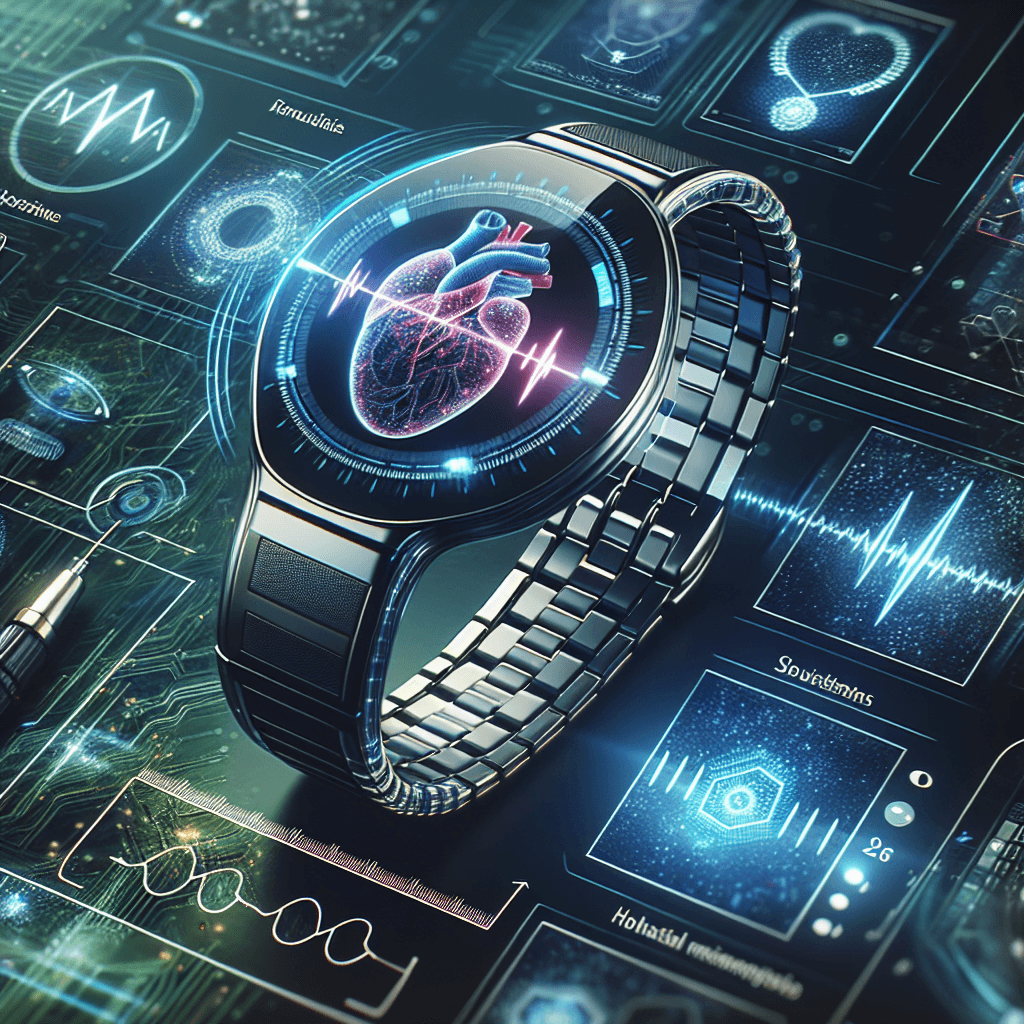
Related site – Digital Twins in Medicine: Pioneering Personalized Treatment Strategies